Project | All projects ↗
Using machine learning as a material to generate and refine aircraft design prototypes
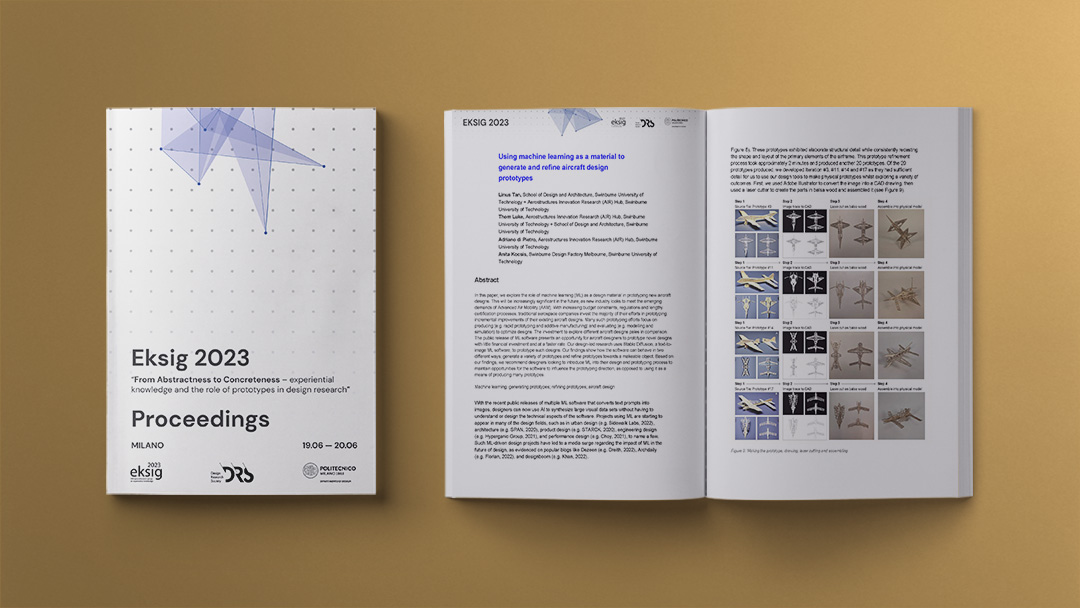
Tan, L., Luke, T., di Pietro, A., & Kocsis, A. 2023. Using machine learning as a material to generate and refine aircraft design prototypes, in Proceedings of Design Research Society: Experiential Knowledge Special Interest Group 2023 – From Abstractness to Concreteness, Milano, Italy, 19-20 June 2023, pp796-813
Details
In this paper, we explore the role of machine learning (ML) as a design material in prototyping new aircraft designs. This will be increasingly significant in the future, as new industry looks to meet the emerging demands of Advanced Air Mobility (AAM). With increasing budget constraints, regulations and lengthy certification processes, traditional aerospace companies invest the majority of their efforts in prototyping incremental improvements of their existing aircraft designs. Many such prototyping efforts focus on producing (e.g. rapid prototyping and additive manufacturing) and evaluating (e.g. modelling and simulation) to optimize designs. The investment to explore different aircraft designs pales in comparison. The public release of ML software presents an opportunity for aircraft designers to prototype novel designs with little financial investment and at a faster rate. Our design-led research uses Stable Diffusion, a text-to-image ML software, to prototype such designs. Our findings show how the software can behave in two different ways; generate a variety of prototypes and refine prototypes towards a makeable object. Based on our findings, we recommend designers looking to introduce ML into their design and prototyping process to maintain opportunities for the software to influence the prototyping direction, as opposed to using it as a means of producing many prototypes.
keywords: machine learning; generating prototypes; refining prototypes; aircraft design
- with: Thom Luke, Adriano di Pietro, Anika Kocsis
- year: December 2022 – June 2023
- for: Design Research Society, Experiential Knowledge Special Interest Group
- download: Article